An unsupervised clustering methodology by means of an improved dbscan algorithm for operational conditions classification in a structure
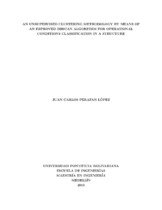
View/Open
Date
2018Author
Perafan López, Juan Carlos
xmlui.dri2xhtml.METS-1.0.item-advisor
Sierra Pérez, Julián
xmlui.dri2xhtml.METS-1.0.item-type
masterThesis
Citación
Metadata
Show full item recordDocuments PDF
Abstract
Structural Health Monitoring (SHM) is highly relevant nowadays, not only for aerospace maintenance, but for a large number of newly engineering applications. Pattern recognition has become an important part of SHM for signal processing and anomalies or damage detection, assuring structural integrity. New methods are created day by day and more researchers and engineers feel the interest to generate techniques which can make SHM become a more compacted, sophisticated and automatized system, eliminating human factors and intrinsic errors. This work evaluates the computational complexity and accuracy of a novel methodology of unsupervised clustering called FA+GA-DBSCAN which employs a combination of machine learning techniques including factor analysis for dimensionality reduction and a density clustering algorithm called DBSCAN enhanced with a genetic algorithm. In order to automatically detect a variety of structural behaviors using the novel methodology an experiment with a beam in cantilever under dynamic loads was taken in consideration.
Keyword/s
Monitoreo de salud estructural
Reconocimiento de modelos
Aprendizaje automático (Inteligencia artificial)
Localización de fallas (Ingeniería)
Algoritmos genéticos
Análisis de datos
Collections
The following license files are associated with this item: